Predictive Analytics & Machine Learning
Traditional approaches to risk adjustment are slow to develop and are often not tailored to the specific casemix of the patients treated by a clinical service. The platform therefore supports a wide range of predictive analytics models and machine learning approaches which enable healthcare organisations to optimise performance using insights contained within their own data.
Metrixcare has developed a range of predictive risk models for areas such as unplanned readmission, surgical site infection, pressure ulcers and falls management. Risk models generated through machine learning features provide analytic tools to perform retrospective risk adjustment of cases that enables appropriate benchmarking and comparison. These risk adjustment models can then be used to move away from reactive historical perspectives towards proactive real-time prediction for harm and waste prevention. For example as soon as a patient is admitted an automated risk prediction for readmission can be calculated. This can be delivered into clinical workflows to highlight patients that may need closer monitoring or specific management to avoid unplanned readmission later.
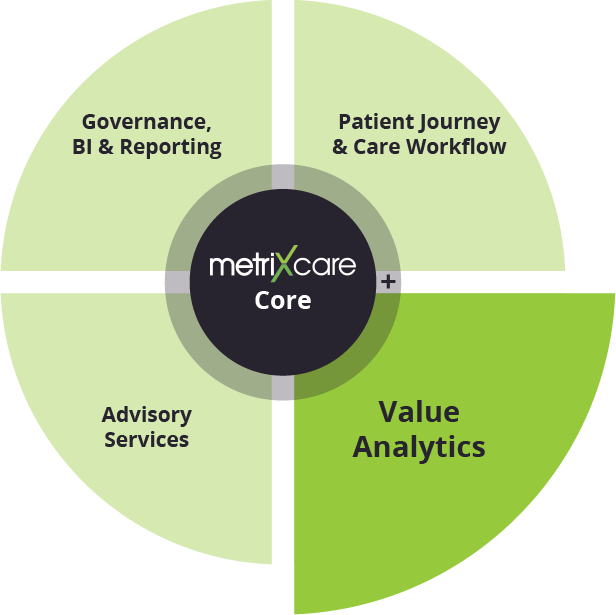
“Optimise performance using lessons learned from your own data”
The Gartner predictive analytics maturity model illustrates the progression from perspectives that provide hindsight through to gaining insights and proactive approaches to managing care.
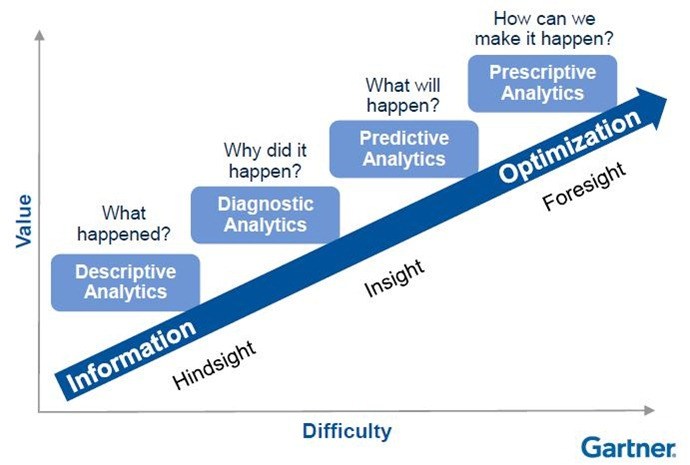
The platform supports supervised and un-supervised learning models that can be tailored to refine the accuracy for your specific patient casemix. Prediction models can be quickly re-trained as frequently as required to adapt to changing operational and clinical needs.